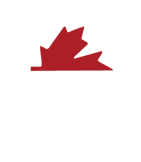
Segmenting Pajar’s Customers with RFM Model Analysis for Better Retention & Growth
RFM Model for an Outerwear Brand
This RFM model was used for one of our long-time clients, Pajar. Pajar is a brand that has been proudly serving its clients with premium winter wear since 1963. With ample experience in the industry, the winter clothing brand understands the harsh realities of winter such as relentless snow and bone-chilling temperatures.
When it comes to providing clients with outerwear to brave the winter elements, Pajar has been known to deliver. Wanting to take their brand to new heights, they searched for a marketing agency that would be able to perform given their personalized requests.
In early Fall 2022, Pajar decided to join Webtmize in the journey of expanding their business across Canada and the United States, and optimizing their paid media campaigns.
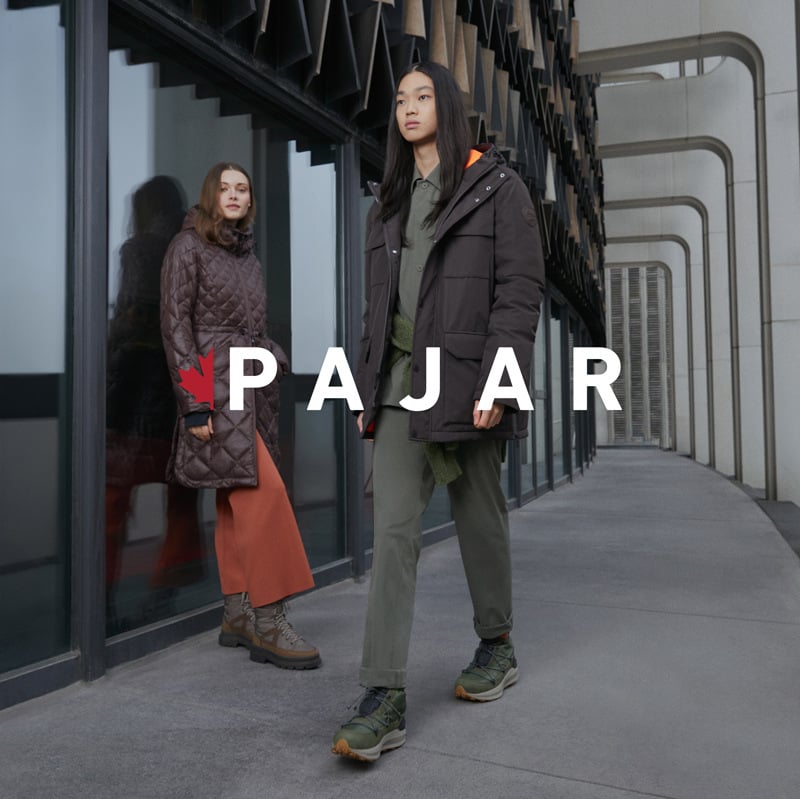
The Challenges That Required an RFM Model
The RFM model analysis for Pajar was no walk in the park. As a highly seasonal brand, Pajar Canada needed to gain a deeper understanding with their eCommerce & in-store customer acquisition and retention. Understanding exactly who their customer base is and how to maximize engagement is crucial to sustaining long-term growth.
The key questions were:
- Who are the high-value customers, and how can they be retained?
- What are the behavioral patterns of different customer segments?
- How can Pajar optimize marketing strategies to increase repeat purchases and customer lifetime value?
- How can Pajar optimize their ad spend and content to effectively engage specific customer segments, maximizing conversion rates?
To address these challenges, we conducted an RFM (Recency, Frequency, Monetary) Analysis and a Cohort Analysis to segment customers, uncover purchasing trends, and provide actionable marketing insights.
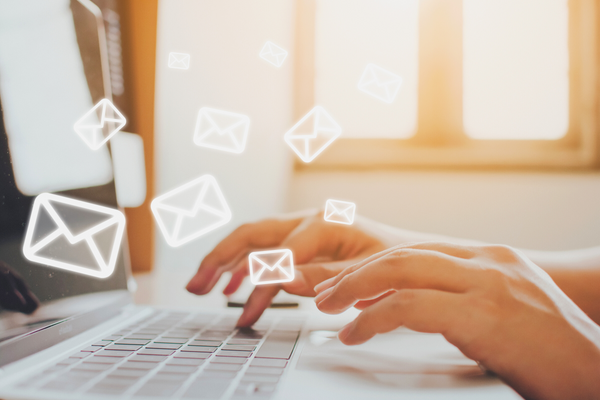
Our Approach: RFM Model Analysis
The methodology of this RFM model for optimizing customer segmentation and retention involved a mix of data extraction, advanced analytical techniques including Machine Learning, and segmentation tools to derive actionable insights. Below is a breakdown of the process, highlighting the role of data science in each phase.
- Data Extraction and Preparation: To gain a comprehensive view of customer behavior, we began by extracting data from Shopify, Pajar Canada’s eCommerce & POS platform. Using Python and SQL, we streamlined the extraction process and ensured the data was structured for analysis. This stage was critical for cleaning and preprocessing the data, which included filtering out ineligible orders, handling missing values, and standardizing data formats.
- RFM Analysis: By calculating the Recency, Frequency, and Monetary scores for each customer, we were able to assess their purchasing behavior and assign each customer a score. By utilizing Machine Learning techniques, we developed a model that automatically classifies customers into four distinct groups: loyal, deal-seekers, casual, and lost shoppers.
- Cohort Analysis: We took a cohort-based approach to analyze how groups of customers behaved over time, particularly those who made their first purchase in a specific month. Cohort analysis allowed us to understand the retention patterns across different customer groups and measure how their purchasing frequency evolved after their initial purchase.
This analysis revealed clear seasonality in customer acquisition and retention, allowing us to optimize marketing strategies around these seasonal shifts.
Key Findings and Insights from the RFM Model
The RFM model analysis revealed four primary customer clusters represented below:
Customer Segmentation via RFM Analysis
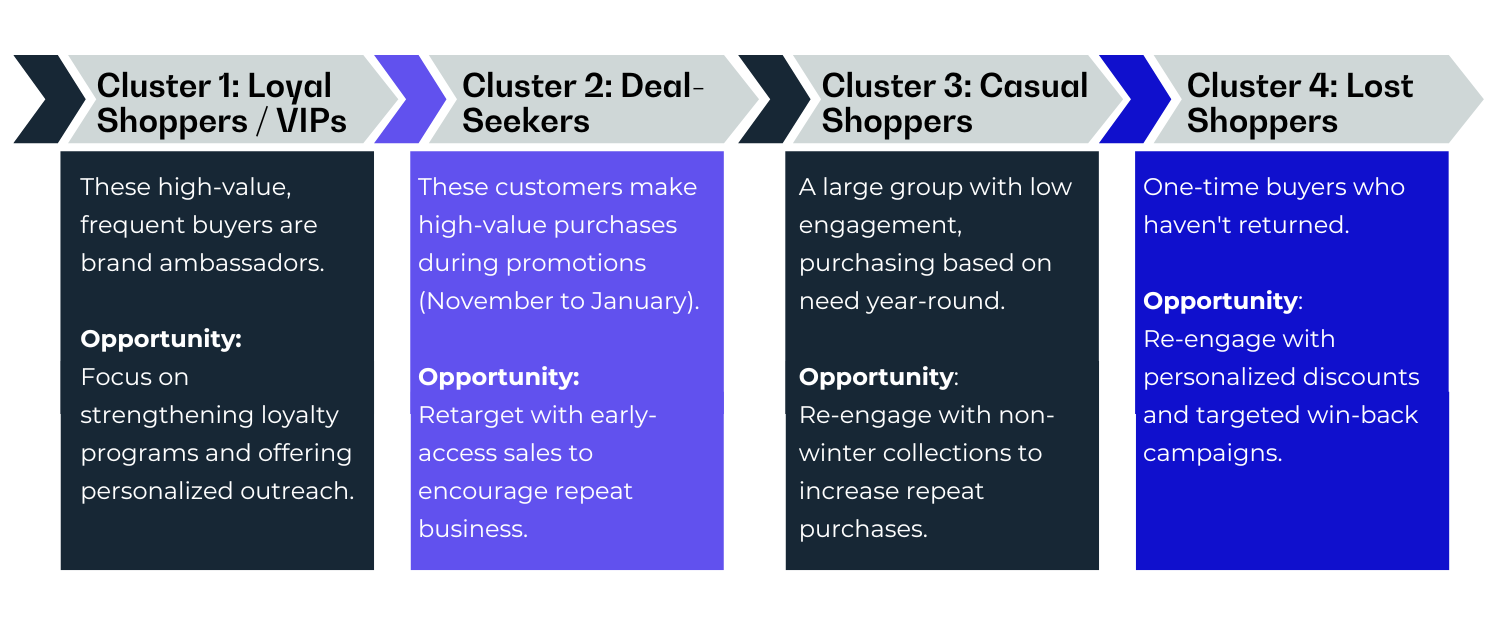
Key Findings and Insights from the RFM Model
The RFM model analysis revealed four primary customer clusters represented below:
Customer Segmentation via RFM Analysis
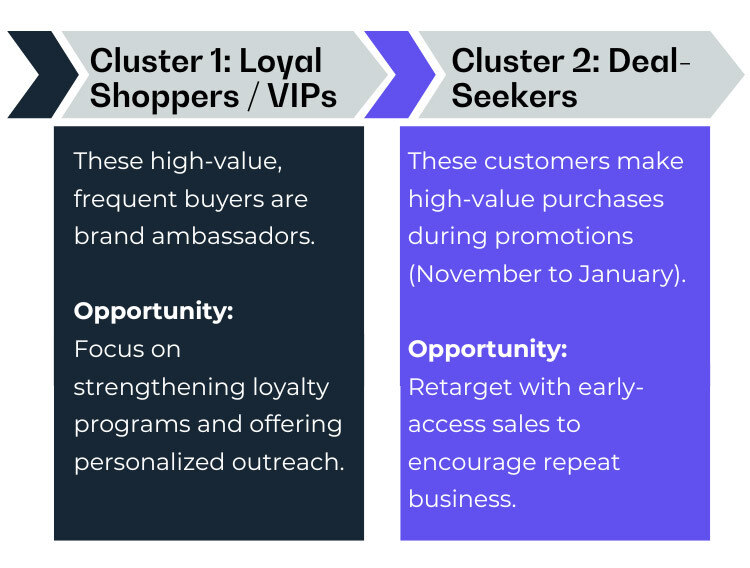
Sales Concentration and Customer Behavior
Pajar's sales distribution closely follows the 80/20 (Pareto) rule, where a small portion of customers generate the majority of sales revenue. The top 30% of customers by lifetime value contribute more than half of total revenue, highlighting Pajar's reliance on a small, high-value segment of its customer base. Within this top tier, Loyal Spenders and Deal Seekers are the primary revenue drivers.
Beyond the top 30%, the remaining customers consist mainly of Casual Shoppers and Lost Shoppers . While their individual contributions to revenue are smaller, their large numbers collectively represent a significant share of Pajar's total sales revenue. Additionally, a substantial portion of Pajar's customers have made only one purchase , underscoring a major opportunity for retention-focused strategies.
Cohort Analysis Insights
Seasonality plays a crucial role in customer acquisition, with notable spikes in November and December and slower acquisition from March to July . Winter 2024 marked the strongest acquisition period in the company's history.
Customer value accumulates over time, as it takes a typical cohort around five years to generate what they contribute in their first month of revenue. This highlights the long-term potential of each cohort and underscores the importance of nurturing customer relationships beyond their initial purchase.
Opportunity: Leverage seasonal trends for optimized retention efforts during peak months and acquisition tactics during non-peak months.
Strategic Recommendations Regarding RFM Model Analysis
Customer Retention Initiatives
- Run Win-Back Campaigns through targeted email marketing to nurture one-time buyers into repeat customers.
- Develop a loyalty program to reward frequent shoppers with discounts and early access.
- Use paid media campaigns to retarget past customers with personalized product recommendations.
Customer Acquisition Improvements
- Increase daily acquisition from 18 to 21 orders through targeted campaigns, potentially boosting yearly revenue by an average of $300K.
- Enhance SEO and content marketing to drive organic traffic.
- Introduce referral programs to encourage customers to invite others.
Seasonal Optimization and RFM Monitoring
- Conduct RFM analysis before each season to refine customer segments.
- Implement flash promotions tailored to specific clusters (VIPs receive early sales access, Deal-Seekers get exclusive discounts).
- Personalize product recommendations based on past purchase behaviour to foster engagement.
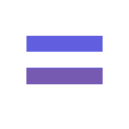
YoY flat
Ad spend
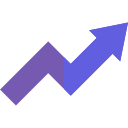
+10%
Net revenue
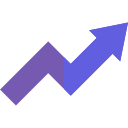
8x
MER
Conclusion
By leveraging RFM and Cohort Analysis, Pajar Canada gained valuable insights into customer behaviour, enabling precise segmentation, better marketing personalization, and increased retention efforts. The strategic recommendations outlined here will empower Pajar to optimize its seasonal business model, drive customer lifetime value, and achieve sustainable growth.
Looking to optimize your customer retention and acquisition strategies? Contact us to learn how our data science expertise can help unlock insights and accelerate business growth.